人工智能在医疗领域的三个时代
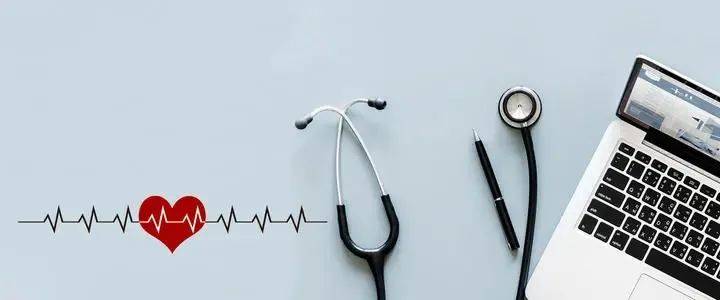
《JAMA》Special Communication
Three Epochs of Artificial Intelligence in Health Care
Ai 汉译仅供参考。
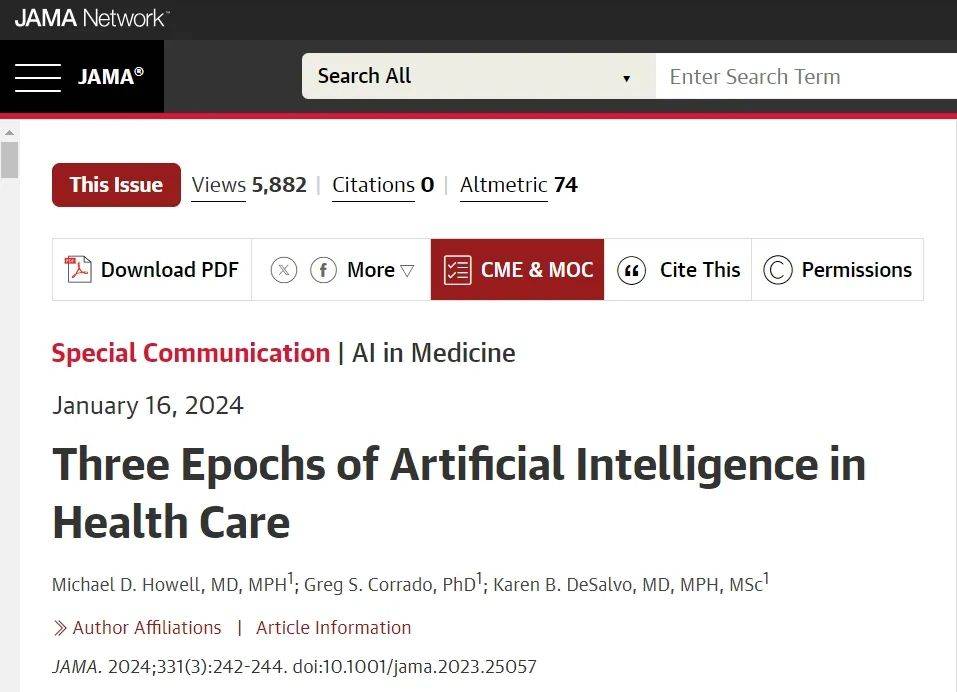
3 Epochs of Artificial Intelligence in Health Care
人工智能在医疗领域的三个时代
Abstract
Importance Interest in artificial intelligence (AI) has reached an all-time high, and health care leaders across the ecosystem are faced with questions about where, when, and how to deploy AI and how to understand its risks, problems, and possibilities.
人们对人工智能(AI)的兴趣达到了历史最高水平,整个生态系统的医疗领导者面临着以下问题:在何处、何时和如何部署AI,以及如何理解其风险、问题和可能性。
Observations While AI as a concept has existed since the 1950s, all AI is not the same. Capabilities and risks of various kinds of AI differ markedly, and on examination 3 epochs of AI emerge. AI 1.0 includes symbolic AI, which attempts to encode human knowledge into computational rules, as well as probabilistic models. The era of AI 2.0 began with deep learning, in which models learn from examples labeled with ground truth. This era brought about many advances both in people’s daily lives and in health care. Deep learning models are task-specific, meaning they do one thing at a time, and they primarily focus on classification and prediction. AI 3.0 is the era of foundation models and generative AI. Models in AI 3.0 have fundamentally new (and potentially transformative) capabilities, as well as new kinds of risks, such as hallucinations. These models can do many different kinds of tasks without being retrained on a new dataset. For example, a simple text instruction will change the model’s behavior. Prompts such as “Write this note for a specialist consultant” and “Write this note for the patient’s mother” will produce markedly different content.
虽然AI作为一个概念自20世纪50年代就存在了,但并非所有的AI都是一样的。各种人工智能的能力和风险存在明显差异,经过考察,人工智能出现了三个时代。AI 1.0包括符号AI,它试图将人类的知识编码到计算规则中,以及概率模型。人工智能2.0时代始于深度学习,在深度学习中,模型从标记有基础真相的例子中学习。这个时代为人们的日常生活和医疗保健带来了许多进步。深度学习模型是特定于任务的,这意味着它们一次只做一件事,它们主要专注于分类和预测。AI 3.0是基础模型和生成AI的时代。AI 3.0中的模型具有根本的新(潜在的变革)能力,以及新类型的风险,如幻觉。这些模型可以完成许多不同类型的任务,而无需对新数据集进行重新训练。例如,一个简单的文本指令将改变模型的行为。提示如“为专科医生写这张纸条”和“为病人的母亲写这张纸条”将产生明显不同的内容。
Conclusions and Relevance Foundation models and generative AI represent a major revolution in AI’s capabilities, ffering tremendous potential to improve care. Health care leaders are making decisions about AI today. While any heuristic omits details and loses nuance, the framework of AI 1.0, 2.0, and 3.0 may be helpful to decision-makers because each epoch has fundamentally different capabilities and risks.
结论和相关性基金会模型和生成AI代表了AI能力的一场重大革命,为改善医疗提供了巨大的潜力。今天,医疗领导者正在就人工智能做出决策。尽管任何启发式都省略了细节并失去了细微差别,但AI 1.0、2.0和3.0的框架可能对决策者有帮助,因为每个时代都有根本上不同的能力和风险。
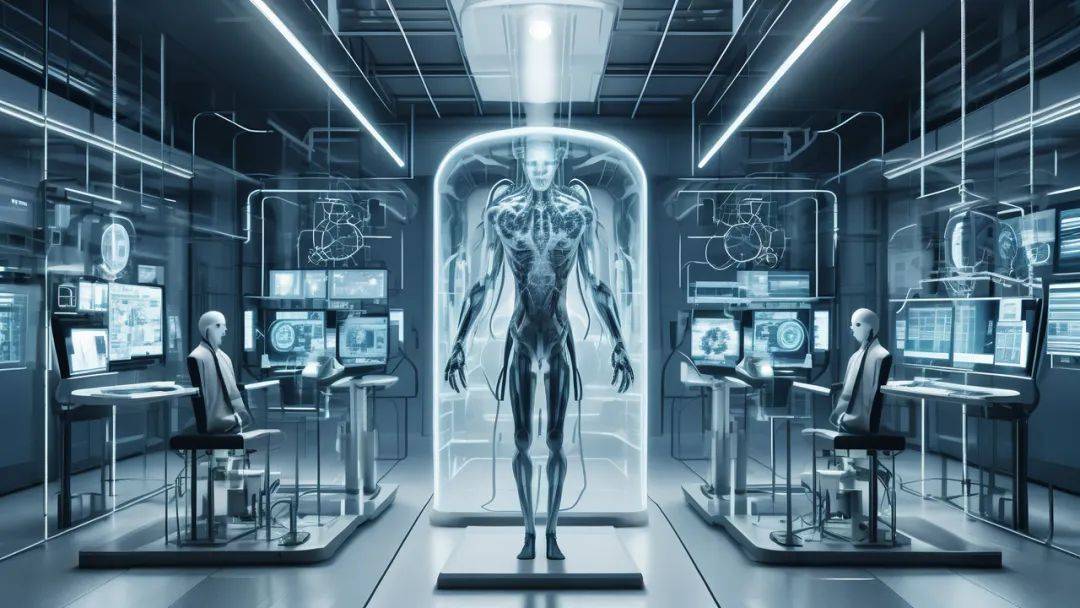
Introduction
Interest in artificial intelligence (AI) has reached an all-time high—whether the metric is scholarly publications, press coverage, or consumer interest. Health care leaders across the ecosystem are faced with questions about where, when, and how to deploy AI and how to understand its risks, problems, and possibilities.
无论是学术出版物、新闻报道还是消费者兴趣,人们对人工智能(AI)的兴趣都达到了空前的高度。整个生态系统的医疗领导者都面临着以下问题:在何处、何时和如何部署AI,以及如何了解其风险、问题和可能性。
All AI Is Not the Same
Capabilities and risks of various kinds of AI differ markedly. Just as grouping bacterial and viral infections together when making a treatment plan could lead to the wrong clinical response, grouping different kinds of AI together may lead health care decision-makers down the wrong path. A simple, pragmatic framework of 3 epochs of AI may assist decision-makers in understanding the strengths, weaknesses, and challenges of different kinds of AI in this moment of technological change (Figure).
所有的人工智能都不一样
各种人工智能的能力和风险明显不同。就像在制定治疗计划时将细菌和病毒感染放在一起可能会导致错误的临床反应一样,将不同种类的AI放在一起可能会导致卫生保健决策者走上错误的道路。一个简单、实用的三个时代的AI框架可能有助于决策者理解在这一技术变革时刻不同类型AI的优势、劣势和挑战(图)。
AI 1.0: Symbolic AI and Probabilistic Models
Over its first 50-plus years, most AI focused on encoding human knowledge into rules in machines. One can think of this as many, many if-then rules, or decision trees. This symbolic AI had some remarkable achievements, such as IBM’s Deep Blue, which defeated the chess world champion in 1997. In health care, tools such as INTERNIST-I aimed to represent expert knowledge about diseases to help with challenging cases. Today, many electronically implemented clinical pathways encode expert knowledge in decision trees, a type of symbolic AI.
AI 1.0:符号AI和概率模型
在最初的50多年里,大多数人工智能都专注于将人类的知识编码成机器的规则。你可以把它想象成很多很多的“如果-那么”规则或决策树。这一具有象征意义的人工智能取得了一些显著的成就,例如IBM的深蓝在1997年击败了国际象棋世界冠军。在医疗保健中,内科医师- i等工具旨在代表有关疾病的专家知识,以帮助处理有挑战性的病例。今天,许多电子实现的临床路径在决策树中编码专家知识,这是一种符号AI。
Symbolic AI also had key limitations, notably a constant risk of human logic errors in its construction and bias encoded in its rules, because its knowledge base depended solely on those creating it. But perhaps the most important issue was that, empirically, symbolic AI had fundamental capability limitations and appeared brittle when confronted with real-world situations. In response, research began focusing more on probabilistic modeling, such as traditional regression and then bayesian networks, which allowed both expert knowledge and empirical data to contribute to reasoning systems. These models handled real-world situations more elegantly and found some use in health care, but in practice were difficult to scale and had limited ability to manage images, free text, and other complex clinical data.
象征性AI也有关键的局限性,特别是在其构建过程中存在人类逻辑错误的风险,以及在其规则中编码的偏见,因为它的知识库完全依赖于创造它的人。但也许最重要的问题是,从经验上看,象征性AI具有基本的能力限制,在面对真实世界的情况时显得脆弱。作为回应,研究开始更多地关注概率模型,如传统回归,然后是贝叶斯网络,这使得专家知识和经验数据都有助于推理系统。这些模型对真实世界情况的处理更优雅,在医疗保健中也有一定用途,但在实践中难以缩放,并且管理图像、自由文本和其他复杂临床数据的能力有限。
AI 2.0: The Era of Deep Learning
Work on even more data-driven methods, broadly called machine learning, was rooted in the idea that key to intelligence was learning from errors. Discoveries such as backpropagation of errors in the 1980s and 1990s laid the groundwork, but in the early 2010s a true revolution happened. As datasets grew and computers sped up, deep learning with multilayered neural networks came into its own and the era of AI 2.0 began. First, the convolutional neural network architecture gave computers the ability to “see,” and they gained the ability to classify images in a photograph (such as “cat” vs “dog”). Second, a discovery known as word2vec created the ability to do math on words at scale.
AI2.0:深度学习的时代
研究更多由数据驱动的方法,也就是被广泛称为机器学习的方法,其根源在于,智能的关键在于从错误中学习。上世纪80年代和90年代的错误反向传播等发现奠定了基础,但在2010年代初,一场真正的革命发生了。随着数据集的增长和计算机的加速,具有多层神经网络的深度学习开始崭露头角,AI 2.0时代开始了。首先,卷积神经网络架构赋予计算机“看”的能力,它们获得了对照片中的图像进行分类的能力(比如“猫”vs“狗”)。其次,一项名为word2vec的发现创造了大规模使用文字进行数学运算的能力。
This revolution changed many things in our daily lives. Today, it’s trivial to search thousands of photographs on a phone without manually labeling each one with what was in them. An individual can even identify things in photographs that they know nothing about, like particular types of plants. Voice recognition is commonplace. One can translate among more than 100 languages, whether by typing or by pointing their camera at words written in a language they do not know.
这场革命改变了我们日常生活中的许多事情。如今,在手机上搜索数千张照片,而不需要手动为每张照片贴上标签,这已经是一件微不足道的事情了。一个人甚至可以在照片中识别出他们一无所知的东西,比如特定类型的植物。语音识别很常见。一个人可以在100多种语言之间进行翻译,无论是通过打字,还是用相机对着用一种他们不知道的语言写的文字。
Deep learning also made new things practical in health care. One of JAMA’s most influential articles of the decade showed ophthalmologist-equivalent identification of diabetic retinopathy in retinal photographs. Researchers also demonstrated breakthroughs in breast and lung cancer screening, pathology, identification of skin conditions, and predictions from electronic health record data, among many other areas.
深度学习还让新事物在医疗领域变得实用。《美国医学会杂志》(JAMA)十年来最具影响力的一篇文章显示,眼科医师可在视网膜照片中发现糖尿病性视网膜病变。研究人员还展示了在乳腺癌和肺癌筛查、病理学、皮肤疾病识别和电子健康记录数据预测等许多领域的突破。
Deep learning algorithms learn from examples labeled with ground truth (“This picture is a cat”). They then learn patterns, rather than being programmed with what the patterns are. In this era, it became easier to program computers to learn than it was to hard-code them with expert-provided rules, at least for many tasks. These models have remarkable capabilities, but also important risks. Models can fail when real-time data differ from the data they are trained on. For example, if a model is trained solely on “cat vs dog” but is given a picture of an airplane, it will not give a good result. More subtle versions of this out-of-distribution problem are a critical safety issue in health care. Complex biases can also arise, related to inclusivity of underlying data, to unequal and unfair diagnostic and therapeutic choices based on race, to algorithmic design choices, and to other issues. Regulators have developed frameworks to assess these types of task-specific AI; for example, the US Food and Drug Administration has approved or cleared hundreds of AI-enabled medical devices.
深度学习算法从标记了基本事实的例子中学习(“这张照片是一只猫”)。然后他们学习模式,而不是按照模式编程。在这个时代,通过编程使计算机学会比用专家提供的规则硬编码计算机更容易,至少对于许多任务来说是这样。这些模型具有非凡的能力,但也有重要的风险。当实时数据与它们所训练的数据不同时,模型可能会失败。例如,如果一个模型只接受“猫vs狗”的训练,但给出的是一幅飞机的图片,它不会给出一个好的结果。这种分配外问题的更微妙版本是医疗保健中一个关键的安全问题。还可能出现与以下因素相关的复杂偏见:基础数据的包容性、基于种族的不平等和不公平的诊断和治疗选择、算法设计选择和其他问题。监管机构已经开发了框架来评估这类特定任务的人工智能;例如,美国食品和药物管理局已经批准或批准了数百种人工智能医疗设备。
AI 3.0: Foundation Models and Generative AI
AI 2.0 had a key problem, related to the somewhat dramatically named catastrophic forgetting: when handling long sequences of text, it had trouble remembering things earlier in the sequence. The transformer architecture that arose in 2017 helped deal with this, letting the model pay attention across long passages. In the following years, transformers were combined with more compute and more data, creating foundation models and large language models. The rate of progress accelerated dramatically in 2022 and 2023, marking the third epoch.
AI 3.0:基础模型和生成AI
AI 2.0有一个关键问题,与灾难性遗忘有关:当处理长文本序列时,它很难记住序列中较早的内容。2017年出现的变压器架构帮助解决了这一问题,让模型能够将注意力放在长通道上。在接下来的几年里,变形器与更多的计算和数据结合在一起,创建了基础模型和大型语言模型。2022年和2023年的进展速度显著加快,标志着第三个时期。
Two key elements differentiate AI 2.0 from AI 3.0. First, AI 2.0 is task-specific. It does one thing at a time. If an individual wants it to do something else, they will need a new dataset and to train a new model. Second, AI 2.0 for the most part predicts things or classifies them. Its ability to generate new words, images, or other content is limited.
区分AI 2.0和AI 3.0的两个关键因素。首先,AI 2.0是针对特定任务的。它一次只做一件事。如果一个人想要它做其他的事情,他们将需要一个新的数据集和训练一个新的模型。其次,AI 2.0在很大程度上可以对事物进行预测或分类。它生成新词、图像或其他内容的能力是有限的。
AI 3.0 is fundamentally different. It can do many different tasks without being retrained. For example, a simple text instruction will change the model’s behavior. A prompt like “Write this note for a specialist consultant” and “Write this note for the patient’s mother” will produce markedly different content. The models also have dramatically improved capabilities: interpreting truly complex questions; accepting and producing text, images, and sound; creating responses that are near-indistinguishable from those written by people; and carrying on extended conversations. There are several types of these models, but for the rest of this section, we’ll focus on an important category—large language models.
AI 3.0有本质上的不同。它可以完成许多不同的任务,而不需要重新训练。例如,一个简单的文本指令将改变模型的行为。像“给专科医生写这张纸条”和“给病人的母亲写这张纸条”这样的提示会产生明显不同的内容。这些模型的能力也有了显著提高:解释真正复杂的问题;接受并产生文本、图像和声音;创造和别人写的几乎没有区别的回复;并进行长时间的交谈。这些模型有几种类型,但在本节的其余部分中,我们将重点关注一个重要的类别——大型语言模型。
They are already impacting our daily lives, in writing assistants, image generators, software-coding assistants, and chatbots. Health-specific large language models also now exist. For example, Med-PaLM and Med-PaLM 2 are medically tuned foundation models, developed at Google, that reached expert-level performance on medical licensing examination–style questions.They were also able to write long-form answers to people’s health questions. When physicians compared Med-PaLM 2’s answers vs those written by physicians without knowledge of the origin, they strongly preferred the model’s answers on 8 of 9 dimensions evaluated.
它们已经影响了我们的日常生活,包括写作助手、图像生成器、软件编码助手和聊天机器人。目前也存在与健康相关的大型语言模型。例如,Med-PaLM和Med-PaLM 2是在谷歌开发的医学调优基础模型,在医师资格考试类型的问题上达到了专家水平的表现。他们还能写出人们健康问题的长篇答案。当医师将Med-PaLM 2的答案与不知道起源的医师所写的答案进行比较时,他们强烈倾向于评估的9个维度中的8个方面的模型答案。
How are large language models trained? Imagine taking a huge stack of documents. A person shows the model each word, in sequence, but does not let it see the next word. Instead, the model is asked to predict the word, again and again. Every time the model gets it wrong, it changes its internal representation of how words fit together. Eventually, it builds a representation of how these words (and thus concepts) fit together. When the model is asked a question later, it responds by predicting likely next words in an answer.
如何训练大型语言模型?想象一下拿着一大堆文件。一个人按顺序向模型显示每个单词,但不让它看到下一个单词。相反,这个模型被要求一次又一次地预测这个词。每当模型出错时,它就会改变单词如何组合在一起的内部表示。最终,它构建了这些单词(以及概念)如何组合在一起的表示。当模型稍后被问及一个问题时,它会通过预测答案中可能出现的下一个单词来做出回应。
Think of the basic versions of these models as next-word prediction engines. This helps make sense of some of their surprising behaviors. For example, these models can be good at writing computer programs, but bad at arithmetic. Why? It is because they are not doing math, they are predicting the next word in a sequence. Similarly, they may return plausible-sounding but incorrect journal citations. Why? For the same reason: they are not looking things up in PubMed, they are predicting plausible next words. These “hallucinations” represent a new category of risk in AI 3.0. This is an area where technical progress in areas such as grounding and in retrieval-augmented generation are actively improving performance, and the ability for these models to use tools like calculators, or have real-time access to the web, also improves results. The bias and equity risks that were present in AI 2.0 remain issues with AI 3.0. In addition, language models may create new kinds of risks because of bias encoded in the semantics of language, among other reasons.
把这些模型的基本版本看作下一个单词的预测引擎。这有助于理解它们一些令人惊讶的行为。例如,这些模型可能擅长编写计算机程序,但不擅长算术。为什么?这是因为他们不是在做数学,而是在按顺序预测下一个单词。同样地,他们可能会返回听起来似是而非的期刊引用。为什么?出于同样的原因:他们不是在PubMed上查找东西,而是预测下一个可信的单词。这些“幻觉”代表了AI 3.0的新风险类别。在这一领域,地面和检索增强生成等领域的技术进步正在积极改善性能,而且这些模型使用计算器等工具或实时访问网络的能力也改善了结果。AI 2.0中存在的偏差和股权风险仍然是AI 3.0的问题。此外,由于在语言语义中编码的偏见,语言模型可能会产生新的风险。
We anticipate that AI 3.0 will come into practice as augmentation tools, initially helping with aspects of health care such as documentation burden. As these tools subsequently begin to support clinical practice, with clinicians in the loop, a thoughtful regulatory framework will be needed to help ensure that patients receive the benefits of this technology safely.
我们预计AI 3.0将作为增强工具投入实践,最初帮助解决医疗保健方面的问题,如文档负担。随着这些工具随后开始支持临床实践,并且临床医师参与其中,我们需要一个经过深思熟虑的监管框架,以帮助确保患者安全地获益于这一技术。
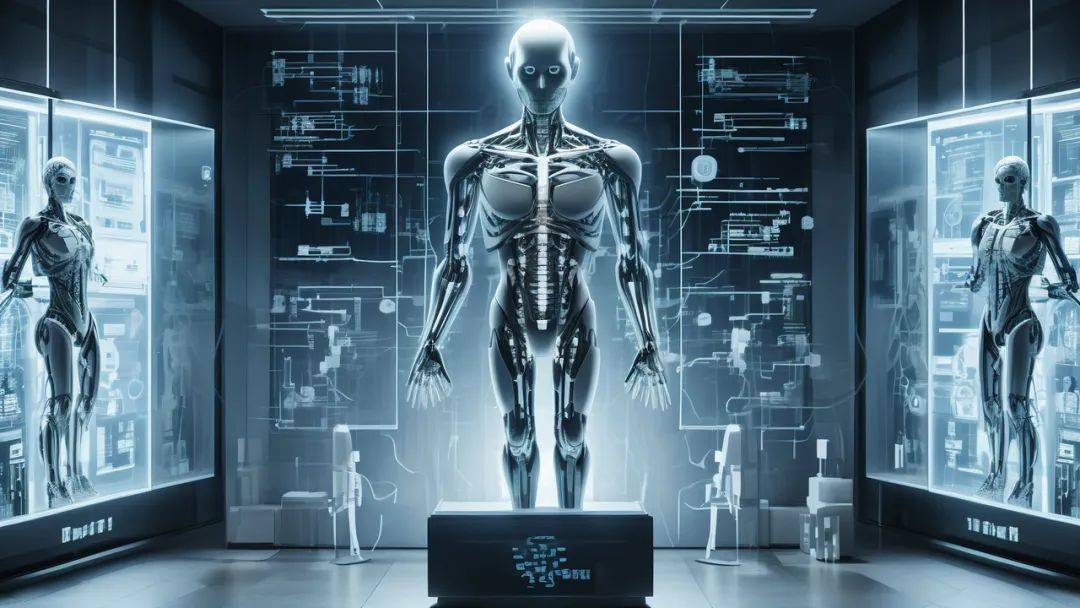
Conclusions
Foundation models and generative AI represent a major revolution in AI’s capabilities, offering tremendous potential to improve care. Health care leaders are making decisions about AI today. While any heuristic omits details and loses nuance, the framework of AI 1.0, 2.0, and 3.0 may be helpful to decision-makers, because each epoch has fundamentally different capabilities and risks.
基础模型和生成AI代表了AI能力的一场重大革命,为改善医疗提供了巨大潜力。今天,医疗领导者正在就人工智能做出决策。尽管任何启发式都省略了细节并失去了细微差别,但AI 1.0、2.0和3.0的框架可能对决策者有帮助,因为每个时代都有根本上不同的能力和风险。
排版编辑:肿瘤资讯-Astrid